Accelerators (Keno Fischer Quora Session)
Keno Fischer, Julia Computing Co-Founder and Chief Technology Officer (Tools) participated in a Quora Session March 18-23.
What role do TPUs and other accelerators play in machine learning?
The success of machine learning has kicked off a bit of a race in the hardware world. It is hard for startups (or even well-funded groups at big companies) to compete in the CPU/GPU chip design world (my understanding is that per-chip development costs there are starting to approach $1 billion). Competing in the machine learning world is a lot easier. You can get away with reduced precision (bfloat16 in the TPU case), increased specialization (e.g. using systolic arrays rather than scalar or vector processors) and to some extent an increased reliance on software to enable simpler designs on the hardware side. I should note that all of these accelerators are still incredibly sophisticated pieces of engineering with development costs in the high 10s to low 100s of millions of dollars range, but that does put them squarely in the range of a large company or well-funded VC-backed startup. Google's TPUs are probably the first of this generation of accelerators to hit the market, but it is quite a crowded space and my understanding is that we'll see a number of additional market entries this year.
I'm generally of two minds about such highly specialized accelerators. On the one hand they provide an enormous opportunity for research and advancement. An accelerator today might be an order of magnitude (sometimes more) cheaper than doing the equivalent computation on a general purpose GPU. That essentially allows you to "time travel" 2-3 years into the future until the general purpose chips have caught up. This is an enormous advantage as it allows trying out new ideas that wouldn't have been possible without special purpose chips.
That said, I do worry that the current generation of accelerators overspecializes to the current generation of machine learning models. Part of the reason machine learning models look the way they do (lots of big matmuls and convolutions, very little dynamic behavior) is that these operations were the only ones supported well by previous generation frameworks (partly for hardware reasons, but often for software reasons that will or have been resolved by current and next generation frameworks). The design of these accelerators may solidify bad assumptions and stifle research into alternatives. I'm hopeful that we'll see a bit of a convergence from both sides. CPUs and GPUs will catch up with accelerators in terms of raw performance (and frankly the V100 is already performance competitive on a chip-by-chip basis for ML workloads, just prohibitively expensive) and the next generation of accelerators will become more flexible.
Another cause for concern with accelerators is that the various vendors will try locking down the ecosystems. CPUs have traditionally had very well documented microarchitectures [1] which allows people targeting them to unlock absolutely peak performance and flexibility. GPUs are quite a bit worse in this regard (AMD is much, much better than NVIDIA - so credit where it is due). NVIDIA does not document the internal instruction set of their GPUs and does not expose it as an interface (instead they provide a virtual instruction set and a tool that does the translation). This prevents anybody other than NVIDIA from writing libraries that achieve peak performance and has (in my opinion) been a significant depressor of innovation in the machine learning world. If accelerators continue on this path and take it even farther, we'll start seeing problems.
TPUs for example are quite locked down at the moment. You used to be able to only run TensorFlow graphs on the hardware. We worked with Google to get a little lower level access at the XLA level (a compiler IR of array abstractions), but even so details about the internal workings of the TPU remain sparse and only people inside Google are able to fully leverage them. I hope the new entrants into the market will take the lessons of history to heart and be as open about the capabilities of their hardware as possible (and I keep encouraging the Google folks to do the same, because I know TPUs are significantly more powerful than I can currently make use of).
[1] There is a severe lack of public documentation around things like the early boot process in current generation processors on both the Intel and AMD side, but that's a separate topic.
We've found Julia to be surprisingly easy to re-target to new backends. I don't think that necessarily had to be the case, so it's worth examining some of the effects at play here that have allowed this to happen.
-
Multiple Dispatch. It's hard to overstate how heavily Julia relies on multiple dispatch for functionality and performance, and it has turned out to be a crucial feature for re-targeting Julia as well. The way we do multiple dispatch in Julia allows us to write algorithms very generically, without much assumption on the underlying execution model of the hardware. This leads to a very high amount of code re-use in the Julia community in general, but in particular allows us to share a huge amount of library code and utilities across backends. Stefan recently gave a talk at JuMP-dev that had a good introduction and explanation of multiple dispatch and its power (video).
-
Contextual Execution. This one's fairly new and we're still figuring out how to do it properly, but the basic idea is to extend multiple dispatch to contextual concerns like "where am I running." For example, multiple dispatch allows alone you to express that "in order to multiply matrices represented by two references to remote GPU memory, schedule this function on the TPU and pass the pointers as arguments," but that presumes that you are semantically running on some host device and just streaming commands to the accelerator. But what if you want to semantically run on the device itself (e.g. because you're the one implementing the matrix multiply)? You could reuse the "matrix in GPU memory" abstraction, but it's not really right because that was supposed to represent a remote handle. Also, the action we defined before isn't quite right anymore. You don't have to schedule anything, you can just call a function. At the very least the backend has to do some rewriting here. A much cleaner approach is to re-use the same data types on both CPU and GPU (e.g. a "matrix" is a pointer to some data and 2-tuple of numbers of rows and columns) and have your dispatch decisions include where you're running as just another dimension to dispatch on (if I'm running on CPU, use this algorithm, if I'm running on GPU use that algorithm). Of course, as usual, you only use this mechanism if there's a good reason to diverge the code paths. If you don't care and the code works anywhere, you can just leave the context unconstrained. In Julia, this kind of thing has been pioneered in the Cassette (https://github.com/jrevels/Cassette.jl) package, but we're slowly moving it closer to the base language.
-
LLVM. LLVM is a fantastic piece of infrastructure and takes care of a lot of heavy lifting. Particularly for backends with similar execution models (different kinds of CPU or even just different micro-architectures of the same CPU family), LLVM papers over a lot of the differences and lets us focus at a higher level in the stack. LLVM will turn "pretty good" IR input into amazing machine code, but it doesn't magically fix language design mistakes for you. I think this is a general property of compiler technology that's often overlooked. Compiler technology is generally multiplicative on the quality of the base language. If you start with an ill-designed core language, all the compiler tricks in the world will get you at best something that's "not quite as bad". But if you start with a sensible language design and particularly if the language is designed to respect the theoretical limitations of the compiler (e.g. making it possible to eliminate abstractions form local information alone), compiler technology can basically achieve wonders.
-
Reusable Compiler. One of the things I think is fairly unique about Julia is that we're able to provide additional backends as packages and don't have to force them into the base language (of course we have the option to do so if there is a good reason). The reason this is possible is that we allow packages to reach into and reuse the internals of the compiler (replacing the parts they need for their particular backend). I'd like to do even more of this in the future and make it better supported. Doing this well will require coming up with some clean APIs and making them more stable (at the moment it's a bit of an informal agreement and the set of users is fairly small), but I think there is a lot of power in compiler technology that users don't make use of, because it would require distributing custom versions of the language to users, which is a prohibitive cost. An example of this kind of thing is our compiler-based reverse mode AD system (FluxML/Zygote.jl), which does a very fancy compiler transform, but is implemented entirely as a library.
-
Type Inference. Type inference is the secret sauce that lets us look like an extremely dynamic language to the user, but at the same time provide LLVM, which is fundamentally a static compiler, with enough static information for it to be able to perform the requisite optimizations. Striking this balance well is a question of careful language design and beyond the scope of this reply. One fun thing about it however, is that this technique is not restricted to using LLVM as the static compiler backend. For example, you can replace LLVM with Google's XLA compiler that generates code for TPUs and get very similar properties (a dynamic look on top of static properties). The kind of static information that XLA needs is quite different from LLVM (XLA reasons about tensors and their layouts, LLVM reasons about data types and memory), but inference doesn't really care about this distinction. This approach is exactly what we did to target TPUs from Julia and it works quite well, particularly in conjunction with some of the other techniques mentioned above. Making this happen, took just a few hundred lines of code (paper: Automatic Full Compilation of Julia Programs and ML Models to Cloud TPUs).
When should one use a CPU, a GPU, or a TPU? Is there a simple example problem that illustrates when one should switch between the devices?
CPUs are general purpose devices, so they're generally the right starting point for most kinds of code. GPUs are getting more general purpose these days also, but they tend to make some different choices in the design space. Among these are:
-
Very wide SIMD units. My recollection is that the SIMD width of a GPU (e.g. a V100) is about 32 (GPU folks tend to call this the warp size, but it's the same concept). For FP64 that's 4x larger than the SIMD width supported by modern Intel CPUs with AVX512 (which have 512 bit wide registers, i.e. a SIMD width of 8 for FP64). The trade-off here is that wider SIMD units save area on the device because you can share common infrastructure (decode, dispatch units, etc.), but of course your program needs to be fairly regular to be able to take advantage.
-
Very high bandwidth memory. GPUs tend to package high bandwidth DRAM relatively close to the chip and thus get significantly higher memory bandwidth than CPUs get to main memory. For example, the V100 has 900GB/s bandwidth to HBM memory. The bandwidth from a CPU to DDR4 memory varies a bit depending on the exact CPU and memory used, but tends to be in the high tens of GB/s. For memory bound applications this can make an enormous difference. Of course the trade-off here is that high bandwidth attachment requires a lot of (short, low noise) wires with chips generally soldered or otherwise bonded right next to the GPU die. As a result, HBM will always have significantly less capacity than main memory would. The biggest GPU you can buy has 32GB of main memory (which has been available for less than a year), but systems with TBs of main memory have been available for more than a decade and even commodity systems can have hundreds of GB of main memory per socket.
-
**Lots of (relatively slow) cores. **This is a bit of a throughput vs latency trade off. Do you build one big core that gets you the answer as fast as possible? Or do you build simpler cores, but lots of them to get to the answer slower, but be able to process more data in parallel?
-
An exposed memory hierarchy. On CPUs, the memory hierarchy (the various levels of caches) is generally automatically managed by the hardware and a lot of complexity goes into making this work well. On GPUs the programmer has to explicitly declare what kind of memory various data goes into. This again makes the hardware simpler, at the cost of putting more load onto the compiler and the programmer
I should note that it is possible to create CPUs that are more GPU-like in their trade-offs. Intel's (now canceled) KNL chip was an attempt to do this. It was the first chip to have AVX512 (though the Xeons have caught up by now). It had onboard HBM (available as either an automatically managed cache or an explicitly managed memory space through a boot-time option) and had up to 72 relatively slow (Atom-derived) cores.
So where does this leave us with respect to workloads? Well, your program will work well if does things that the above tradeoffs were designed for. Is your program very regular and does lots of floating point math? Well, the wide SIMD units will probably work well for you. Do you need to access memory very, very quickly (but not too much of it)? The memory bandwidth might help you. Other things (e.g. lots of pointer chasing) will probably be quite slow. Between these two extremes, it will heavily depend on the workload. Of course, there's also always the option of splitting your workload between the CPU and the GPU, though at that point the communication costs can quickly become prohibitive (though I'm hoping the newer generations of PCIe will help a lot here).
Alright, so much for GPU. Now what about TPU? Unfortunately, there is not a huge amount of public information out there and even having run a fair amount of code on the device, I still don't have a great sense of what kind of things will work well. In some dimensions a TPU is quite similar to a GPU (HBM memory, relatively slow cores optimized for throughput). It even has a vector unit that I assume is fairly GPU like (but that's an educated guess). The biggest difference between a TPU and a GPU is that the TPU has a matrix unit that does matrix multiplies (and to my understanding some related operations) in hardware, rather than building them out of more primitive vector operations. This leads to efficiencies on the hardware design front, but again makes the software design harder. Another big difference is that the matrix multiply unit is using half precision arithmetic (using a custom floating point format), though GPUs are also adding half precision (and even lower precision) arithmetic to support the machine learning craze.
For workloads on TPU, I think the jury is still out. Obviously standard machine learning models (deep neural networks, etc.) will work pretty well, but beyond that it's not quite clear. Since it shares so many characteristics with GPUs starting from those kinds of workloads makes a lot of sense, but to get good performance out of the TPU, you really do want to load the matrix units. Another problem with the TPU is that the software stack is currently limiting the capabilities of the hardware. For example, on GPUs you can easily page out memory from HBM into main memory until you need it again. This is possible hardware wise on TPUs also, but there isn't really a great way to specify that in software. I think we'll find out over the next year or so what works well on TPU. If you have ideas, particularly for Julia workloads that could work well - do let me know. I'm trying to figure it out myself.
About the Author
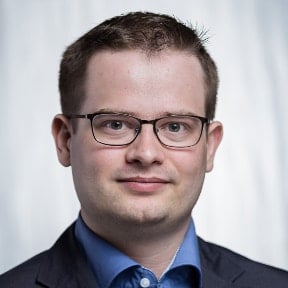